Q&A: Evaluating AI Tools for Healthcare With Northwestern Medicine

Artificial intelligence has been the main topic of discussion at major healthcare technology events so far this year, such as ViVE and HIMSS.
During the 2025 HIMSS Global Health Conference and Exhibition in Las Vegas, industry leaders shared targeted AI use cases and offered insights into promising solutions for clinical workflows.
“We’re seeing more effective pilot programs that generate real-world evidence, which helps to enhance the ability for AI to impact patient care or other operational efficiencies,” says Hannah Koczka, vice president for NM Ventures and Innovation at Northwestern Medicine.
She spoke to HealthTech following HIMSS about how her team identifies and deploys AI solutions, finding the right partnerships, and prioritizing open communication across an organization.
Click the banner below to read the new CDW Artificial Intelligence Research Report.
KOCZKA: First, we try to understand what our requirements and objectives are, finding the specific challenges or areas where AI can add value. At Northwestern Medicine, our focus is in three key areas: improving patient outcomes, streamlining operations and enhancing diagnostics. Once we’ve identified those challenges and the sub-areas within, we’re researching solutions and investigating the various AI solutions available to see which ones align with our identified needs.
At Northwestern Medicine, we also have our own AI development teams, so before bringing in any external AI solution, our team is assessing that it’s not something that has duplicative functionality with something else that NM has already implemented. If it is determined that a solution is a fit, we’re then assessing for data integration feasibility and ensuring any necessary regulatory compliance.
Our team will also evaluate how easy it will be for staff to use the AI and how well it can be integrated into current workflows without causing disruptions that would be detrimental. It is also essential for us to understand the financial implications against the anticipated benefits.
HEALTHTECH: How does your organization tackle AI and data governance? Are they separate approaches, or are they connected?KOCZKA: They’re connected, but they’re different groups that are evaluating AI and data governance. Initially, we handle AI governance from a more technical standpoint. Then, if the solution meets those checks, we move on to our data security and privacy teams, architecture and all of those other reviews. We approach an AI technology like we’d approach any software solution that we want to bring into the organization.
Our innovation team tries to find the right environment to pilot and test out those AI solutions in a more controlled space before allowing for a broader rollout. We collect feedback and monitor and evaluate those solutions once they’re more fully deployed across the organization as well.
EXPLORE: How can healthcare organizations overcome AI implementation hurdles?
HEALTHTECH: How important is it for AI solution partners to have healthcare experience? What are your criteria for partnerships?KOCZKA: A partner with healthcare experience is crucial, and I think there are several reasons why. One would be industry knowledge: Healthcare is such a complex build that has unique regulatory requirements and workflows. Vendors need to understand those intricacies, and then they can develop solutions that address those more effectively.
When we look at compliance and regulations, vendors that are familiar with our industry standards, such as HIPAA, can often ensure that those solutions meet requirements. If they have healthcare expertise, they have experience in integrating their products with existing healthcare technologies, which leads to a smoother and faster deployment of the technology.
Proven success is also an essential factor. They should provide us with case studies or references that demonstrate the efficacy and reliability of their solutions within a healthcare context. Also, solutions that can be customized for our use are also essential.
We identify key partners through networking and just hearing what’s out there in the market. Our team tries to stay plugged in through events such as HIMSS and ViVE and via seminars. We’re grateful for our many peer exchanges, which often lead to valuable insights, and accessing market research. Industry reviews and recognitions are also factors that our team considers.
Our team also assesses how long a partner has been in the market and the types of other healthcare organizations they’ve worked with. I would say we bring in a pretty good mix of AI solutions, from established industry players to startup companies. We like to be first to market, or to help startups build their products in our environment.
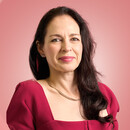
Hannah Koczka Vice President for NM Ventures and Innovation, Northwestern Medicine
KOCZKA: At Northwestern Medicine, our structure is to have cross-functional teams evaluate solutions, developing from the outset a team that includes representation from relevant clinical areas, IT, operations and admin, which encourages diverse perspectives and knowledge sharing. It is fundamental to clearly articulate the goals of the AI solution and how it’s going to benefit each department or stakeholder that could be impacted by its use.
If a solution is brought in, we pilot it pretty quickly and try to have pilot groups from different departments that will test that AI solution in a controlled environment. Having more hands-on experience with the technology really helps with building that collaborative nature and process for the project.
Then, our team will track whether pilots should keep moving forward or whether they should pull back. We offer good channels for open communication for feedback so that we can make quick adjustments. It’s important to involve those users early on and get their input on requirements and workflows. I think it’s like any project that you’re trying to implement.
Our expectations is that our partners demonstrate the technology, showing real-world examples of how the AI solution can either simplify a task, improve efficiency or enhance patient outcomes. It is vital to also celebrate the early successes, which I think helps to build wider adoption over time.
Northwestern Medicine has a robust executive governance process for evaluating all technology solutions, and that includes AI. Our executive committee is multidisciplinary, with finance, operations, IT, compliance and clinical areas represented. They’re typically not necessarily stakeholders, and may or may not use the technology, but they help us to vet our solutions and truly understand cost-benefit analysis. They’re looking at how the solution aligns with our strategic goals, whether it will drive operational efficiency, things like that.
The executive piece comes a bit later in our process, because we may not have a successful pilot, or the users decide the solution isn’t something that works for them. So, we bring our executive team in at the end if there is a request to more widely deploy the technology.
HEALTHTECH: What advice do you have for other healthcare organizations looking to address user concerns early on?KOCZKA: Open communication is key. We try to be very transparent around what our goals are, the processes, the implications of the project, making sure that stakeholders are aware of what to expect throughout the implementation and while using the technology.
It’s crucial to keep stakeholders involved and engaged from the outset of the project and make them feel valued and heard. We'll often have advisory committees, which depends on the size of the project and how many will be impacted across the organization. Those committees can provide ongoing feedback and guidance.
Education and training is another critical area, so we use the vendor’s resources to explain the new technology and its benefits and bring in our own technical and architecture teams to explain how this can integrate with current workflows.
Also, it’s important to address misconceptions about the AI technology. We often show real-world examples and emphasize that AI is intended to enhance human roles. If we’re not the first to use the technology, we show examples of how that AI has positively impacted other organizations and focus on how that allows for improved patient care and operational efficiencies.
KOCZKA: Maintaining data privacy and security is a big one, especially in the clinical space. We’re looking at very sensitive patient data, and we need to ensure compliance with HIPAA. Those things are complicated but obviously paramount before we do anything else.
Data quality and standardization remains another persistent challenge. AI technologies rely on high-quality, standardized data for effective learning and performance, and inconsistencies in data formats and quality across different healthcare systems can hinder AI development or output. Integrating within existing technologies is also complex and resource-intensive, but if you don’t do that, you’ll have interoperability challenges, especially on the clinical side.
Understanding the clinical efficacy and safety of those AI solutions is also important, so we want to ensure that there have been robust validation studies and real-world evidence, which is quite frankly still a significant hurdle for many of these technologies.
Overcoming bias is another issue. We know that AI technologies can either perpetuate or exacerbate existing biases if they aren’t trained on nonrepresentative data, and so we talk a lot about the diversity of our patient base when we’re developing our own AI technologies, which then helps with the diversity of your data and can mitigate those types of issues. It can be hard when you’re bringing in external AI technologies, because you’re not always sure of how those algorithms were trained, and you try to avoid disparities in care. Sometimes, that may mean retraining the AI algorithm on your data.
LEAD MORE: Build a smarter AI strategy for your organization.
User acceptance and adoption are still issues. As in many industries, you’re going to have professionals who might be skeptical or resistant to using these technologies. At Northwestern Medicine, we obviously don’t want to complicate clinical decision-making, so ensuring quality and clinical validation to help gain trust will lead to successful adoption.
I think there’s still regulatory uncertainty. The landscape for AI in healthcare is still evolving. We’re seeing different regulations come out of the U.S. Food and Drug Administration, and those things will probably change. That makes it more challenging for more AI solutions to gain access to the market and to get those technologies into healthcare systems.
Having said all of that, I do think we’re seeing resource groups that allow for more enhanced and easier access to quality data, which then improves AI training. There are advancements that have led to more sophisticated algorithms capable of analyzing large, complex healthcare data work streams, which then leads to improved diagnostic accuracy and predictive capabilities.
There are also numerous consortiums now, which is increasing collaboration among different organizations and helping to foster innovation. Some of those groups come out of big industry players, or healthcare providers are forming their own.
healthtechmagazine